in their debut ML Perf industry-standard AI benchmarks, NVIDIA H100 Tensor Core GPU It sets world records for inference on all workloads, delivering up to 4.5x performance improvement over previous-generation GPUs.
The results show that Hopper is the top choice for users looking for maximum performance with advanced AI models.
moreover, NVIDIA A100 Tensor Core GPU and the NVIDIA Jetson AGX Orin The AI-powered robotics module continued to provide overall leadership reasoning performance on all MLPerf tests (image and speech recognition, natural language processing, and recommender system).
H100, aka Hopper, has raised the bar for performance per accelerator on all six neural networks in the round. It demonstrated leadership in both throughput and speed in individual server and offline scenarios.
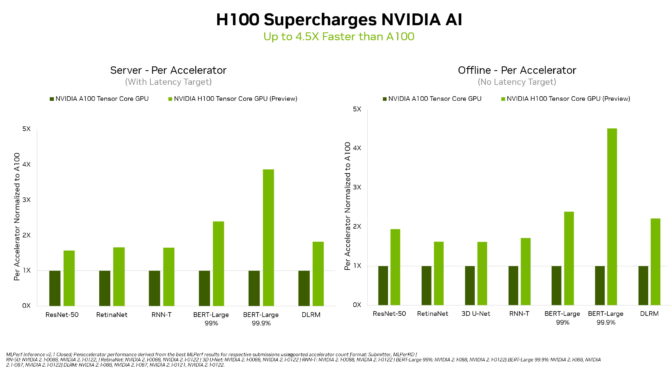
The NVIDIA Hopper architecture outperforms NVIDIA Ampere architecture GPUs by up to 4.5x and continues its overall leadership in MLPerf results.
Thanks in part to the Transformer Engine, Hopper excelled at general BERT models in natural language processing. It is one of the largest and most performance-hungry MLPerf AI models.
These inference benchmarks are the first public demonstrations of the H100 GPU, available later this year. The H100 GPU will join his upcoming MLPerf round for training.
A100 GPU Shows Leadership
NVIDIA A100 GPUs, now available from leading cloud service providers and system manufacturers, continue to demonstrate overall leadership in AI inference mainstream performance in the latest tests.
The A100 GPU scored more tests in data center and edge computing categories and scenarios than any other submission. In June, A100 also demonstrated overall leadership in his MLPerf training benchmarks, demonstrating its capabilities across AI workflows.
Since debuting at MLPerf in July 2020, A100 GPUs have delivered a 6x performance boost thanks to continuous improvements in NVIDIA AI software.
NVIDIA AI is the only platform to run all MLPerf inference workloads and scenarios in data center and edge computing.
Users want versatile performance
The ability of NVIDIA GPUs to provide leadership in all major AI models makes users a true winner. Their real-world applications typically employ many different types of neural networks.
For example, an AI application might need to understand a user’s voice requests, classify images, make recommendations, and deliver responses as voice messages in a human voice. Each step requires a different type of AI model.
MLPerf benchmarks cover these and other common AI workloads and scenarios such as computer vision, natural language processing, recommendation systems, and speech recognition. This testing ensures that users get reliable and flexible deployment performance.
Testing is transparent and objective, allowing users to make informed purchasing decisions based on MLPerf results. Benchmarks are backed by a wide range of groups including Amazon, Arm, Baidu, Google, Harvard, Intel, Meta, Microsoft, Stanford, and the University of Toronto.
Olin leads on edge
For edge computing, NVIDIA Orin runs all MLPerf benchmarks and passes more tests than any other low-power system-on-chip. It’s also up to 50% more energy efficient compared to when it debuted in his MLPerf in April.
In previous rounds, Orin ran up to 5x faster than previous generation Jetson AGX Xavier modules and was on average 2x more energy efficient.
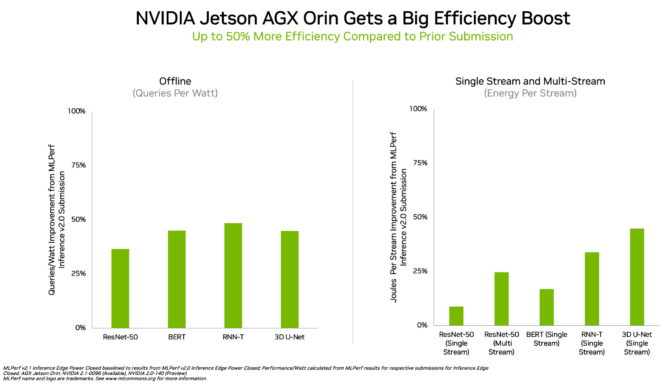
Orin is integrated into a single chip, NVIDIA Ampere Architecture A cluster of GPUs and powerful Arm CPU cores.it is available today NVIDIA Jetson AGX Orin It offers developer kits and production modules for robotics and autonomous systems, and supports the full NVIDIA AI software stack, including platforms for autonomous vehicles (NVIDIA Hyperion), Medical equipment (Clara Holoscan) and robotics (Isaac).
Extensive NVIDIA AI Ecosystem
MLPerf results show that NVIDIA AI is powered by the industry’s broadest machine learning ecosystem.
Over 70 submissions in this round ran on NVIDIA platforms. For example, Microsoft Azure submitted the results of running his NVIDIA AI on their own cloud service.
In addition, 19 NVIDIA Certified System Ten system manufacturers participated in the round, including ASUS, Dell Technologies, Fujitsu, GIGABYTE, Hewlett Packard Enterprise, Lenovo and Supermicro.
Their research shows that users can get great performance with NVIDIA AI both in the cloud and on servers running in their own data centers.
NVIDIA partners participate in MLPerf because they know it is a valuable tool for customers evaluating AI platforms and vendors. Results from the latest round show that her NVIDIA platform will boost the performance it currently offers to users.
All the software used for these tests is available from the MLPerf repository, so anyone can get these world-class results. Optimizations are continuously collapsed into containers available in . NGC, NVIDIA’s catalog of GPU acceleration software.also found there NVIDIA TensorRTused to optimize AI inference across all submissions in this round.
For more information, read our technical blog. Technologies Driving MLPerf Performance from NVIDIA.